Globe Street Tech
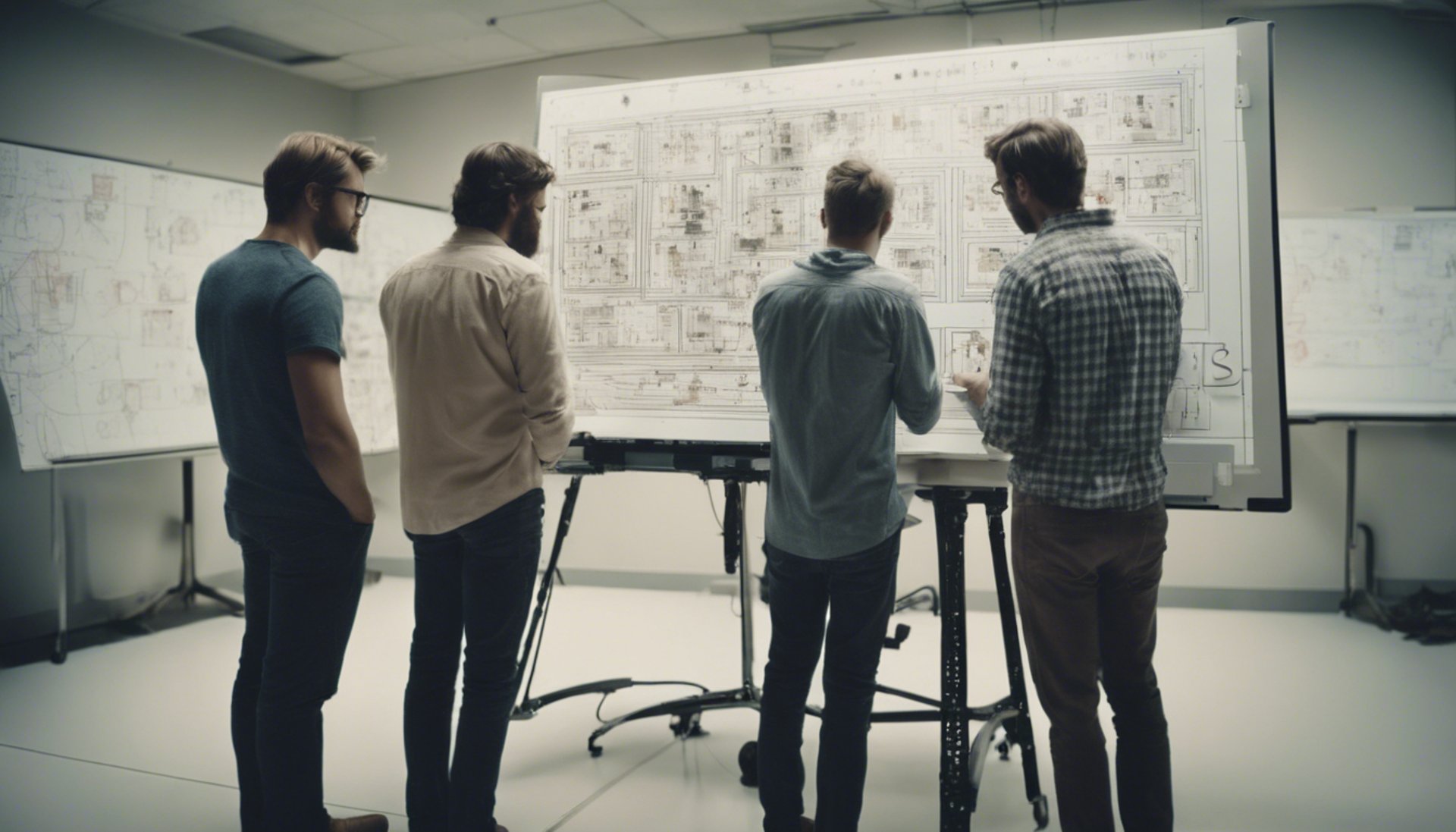
Unleash the Power of Generative AI Systems in your Enterprise
#SemanticRAG
Through 2025, at least 30% of genAI projects will be abandoned...due to poor data quality, inadequate risk controls, escalating costs, and/or unclear business value
Gartner Research - 10 Best Practices for Scaling Generative AI across the Enterprise, Arun Chandrasekaran
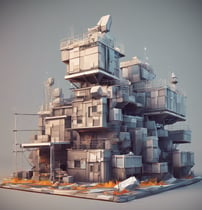
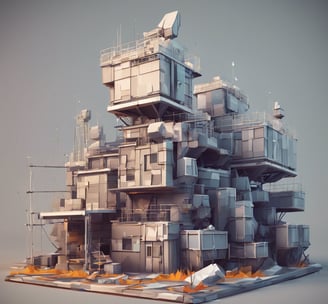
Empowering Your AI Journey
The world has been fundamentally disrupted by the changes generative AI and large language models (LLMs) have brought to life—and businesses are increasingly recognizing the significance of this paradigm shift. Leveraging AI in business provides many benefits, including better decision making, faster time to market, enhanced customer experience, more efficient operations and business growth.
Businesses exploit the opportunities of AI to:
Quickly and accurately analyze and interpret vast amounts of data
Automate business workflows and processes
Reduce human error in data-processing activities
Gain valuable insights to streamline their decision-making processes
Accelerate research and development endeavors
With the implementation of AI, companies can interpret and act upon their data by embedding LLMs in their business-critical applications and systems. Training AI models with enterprise data can lead to greater insights and accurate predictions simply because others don’t have access to this same data.
Proprietary data is unique and exclusive to companies, and exploiting its capabilities can provide a competitive advantage to businesses. Companies with access to confidential or sensitive information, such as healthcare companies legally allowed to use medical records, have a competitive advantage when building AI-based solutions
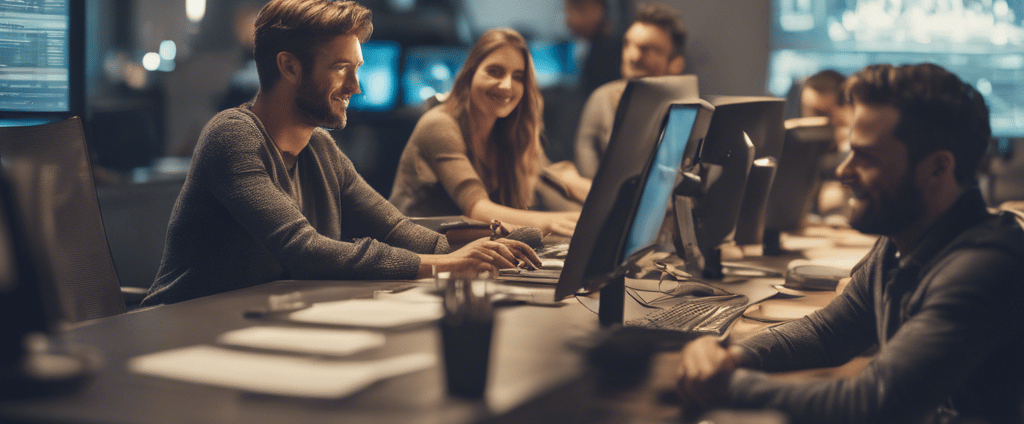
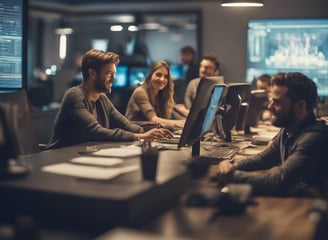
Generative AI : Do more with your data
Risks and Challenges: Enterprise Data and AI
Hallucinations
A hallucination happens when AI reports error-filled answers to the user. In human terms, these are like memory errors or lies. Even though these error-filled answers do sound plausible, the information may be incomplete or altogether false. For example, if a user asks a chatbot about the average revenue of a competitor, chances are those numbers will be way off. These kinds of errors are a regular occurrence. The rate of hallucinations that ChatGPT experiences varies between 15% and 20%, which should be considered when querying your AI (Datanami, 2023).
Fairness/Biases
Data bias impacts day-to-day businesses and occurs when the available data fails to represent the entire population of the phenomenon. This diminishes the fairness and equity of the systems as they may produce results that reflect the biases encoded in the training data rather than present objective reality.
Reasoning/Understanding
Even though LLMs demonstrate exceptional natural language abilities, they are struggling with logical reasoning and understanding of complex concepts. Certain queries that are common sense for human beings may confuse LLMs as they are missing this information in the training data. LLMs can lead to stereotypical or incorrect answers if not carefully monitored and trained
Data Cutoffs
Data cutoffs present a major challenge for LLMs, impacting the model’s ability to accurately understand and respond to users’ queries. Considering the extensive time required to train the model, its memory can quickly become outdated. When LLMs have limited access to information, they may produce answers that do not incorporate recent trends and developments, affecting its accuracy and relevance.
Explainability
How LLMs generate responses can be questionable. They should be trained or prompted to show their reasoning and reference to the data they used to construct a user’s response. A traceable AI-generated response will enhance users’ trust in the model and provide accountability.
Semantic RAG (Retrieval Augmentation Generation)
RAG - Grounding LLM's with your data
Retrieval Augmented Generation allows companies to optimize LLMs’ answers with relevant information without modifying the model. This RAG architecture allows users to apply their enterprise data to their prompt by connecting their query to the knowledge model built and managed in this solution, effectively acting as long-term memory for the generative AI. This provides much-needed context and insight to decrease the chances of hallucination, improve accuracy and reduce costs. By leveraging RAG with proprietary data, users can:
Enhance data privacy with internal data control
Customize the model according to their business needs
Improve accuracy with and reduce hallucinations
Control the content generation and achieve data transparency
Achieve consistent results and trust with the LLM model
Semantic Tags
Using a semantic platform, tag your queries against this knowledge graph and identify relevant content within your data, relating to the subject and context of the queries.
Ingest Content
Ingest content as-is into a multi-model database, curate and harmonize it into the relevant model required for both the LLM and other downstream applications, making it extensible for future applications and services.
Augmented Prompt
Create a prompt using our Augmented Prompt Generation approach, including a hybrid search against the semantic knowledge graph, increasing relevancy and accuracy for the user.
Fact Extraction
Semantically tag your private content using classification based on ontologies, taxonomies, entity and fact extraction— all within a semantic platform that helps you turn your content into a knowledge graph of your data
Refined Query
Pass this prompt to the generative AI and get an answer that is then re- validated against the knowledge graph and the reference documents to promote accuracy
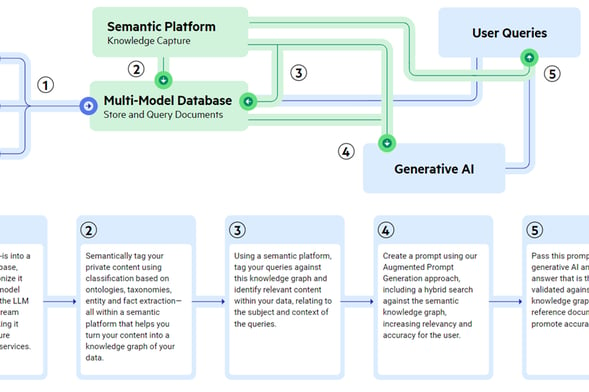
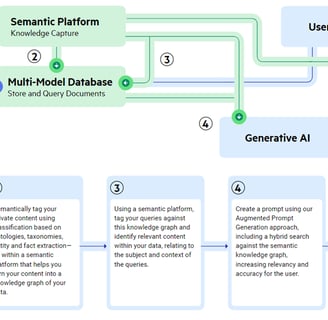
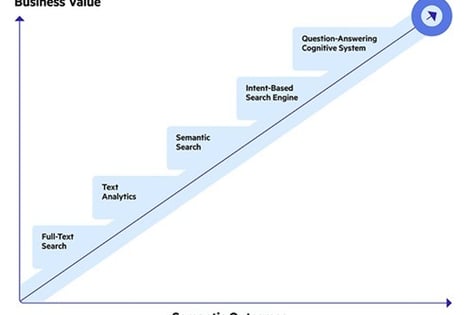
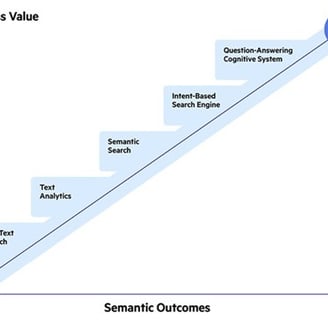
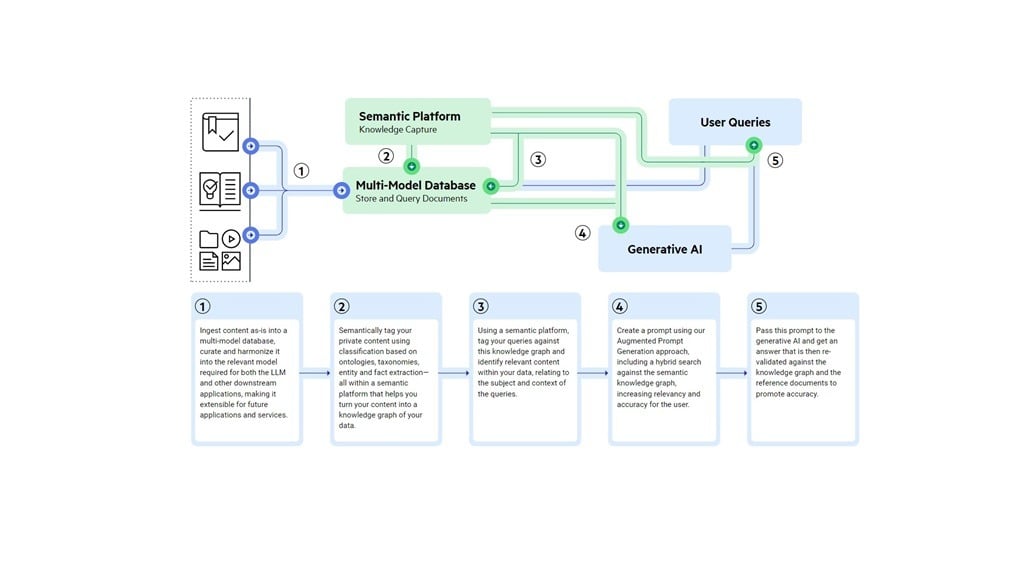
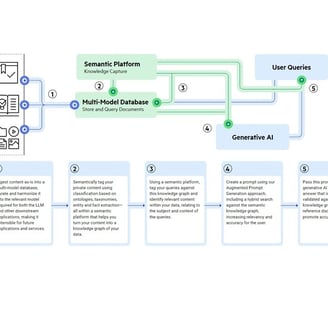
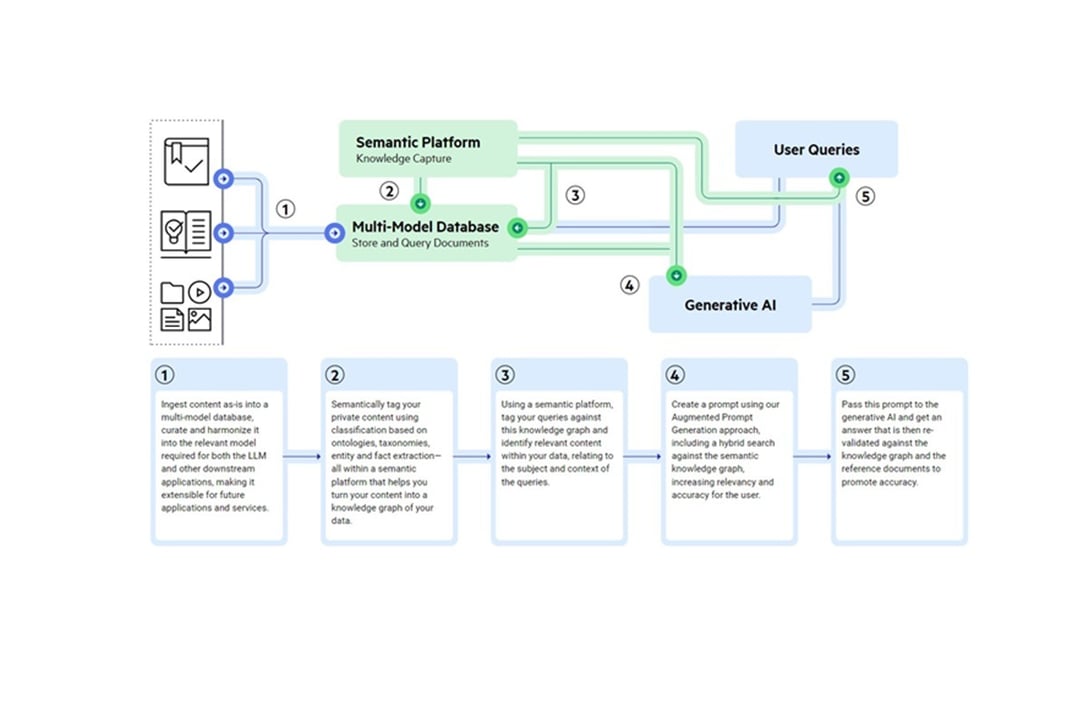
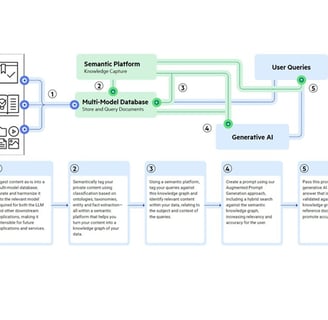
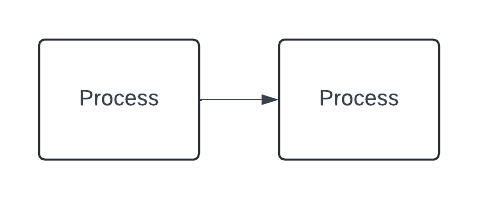
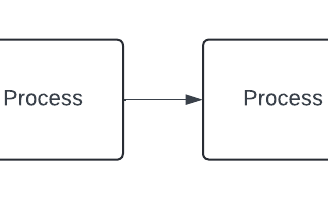
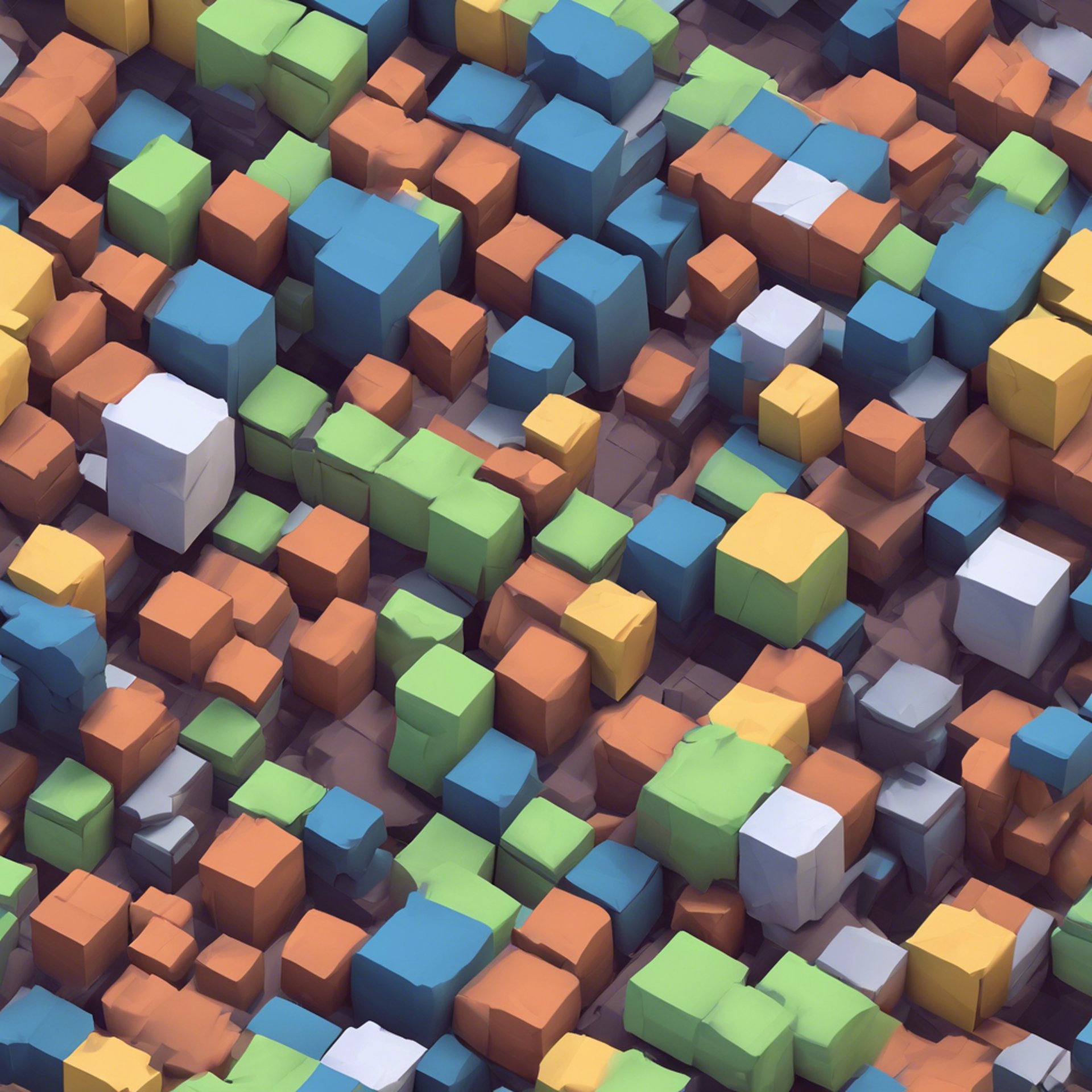
AI Won't Replace Humans - but Humans with AI will Replace Humans without AI
Harvard Business Review
Get in touch
Share with visitors how they can contact you and encourage them to ask any questions they may have.
CONTACT
info@globestreet.com
© 2024. All rights reserved.